
- #Nvidia geforce gt 750m driver update mac how to
- #Nvidia geforce gt 750m driver update mac for mac os x
- #Nvidia geforce gt 750m driver update mac mac os x
#Nvidia geforce gt 750m driver update mac how to
I didn’t have to install Xcode because I have it installed already, but here is a tutorial on how to do it. Install Xcode and native command line tools The first two requirements are met at this point lets get to the last two.

the Clang compiler and toolchain installed using Xcode.
#Nvidia geforce gt 750m driver update mac mac os x
#Nvidia geforce gt 750m driver update mac for mac os x
You can find the installation steps for Mac OS X here. Go to this URL and download the latest version. There are options to install the driver when you install the CUDA Toolkit 8.0, but I preferred to install the driver first, to make sure I have the latest version. Now you have hardware support confirmed, let us move forward and install the driver. Then you need to see if the card is supported by CUDA by finding you card here: In my case, it is NVIDIA GeForce GT 750M. Go to “About This Mac,” and get from there: And the journey begins! Check you have a CUDA GPU card with CUDA Compute Capability 3.0 or higher.įirst, you need to know your video card. It doesn’t mean this is the only way to do it, but I just want to let it rest somewhere I could find it if I needed in the future, and also share it to help anybody else with the same objective. This article will describe the process of setting up CUDA and TensorFlow with GPU support on a Conda environment. It worth trying to have it done locally if you have the hardware already. Nevertheless, I could see great improvements on performance by using GPUs in my experiments. I don’t know about you, but this is a long list to me. When all of that is installed and checked, TensoFlow with GPU support could be installed. ( Compute Capabilities version identify the features supported my the GPU.)
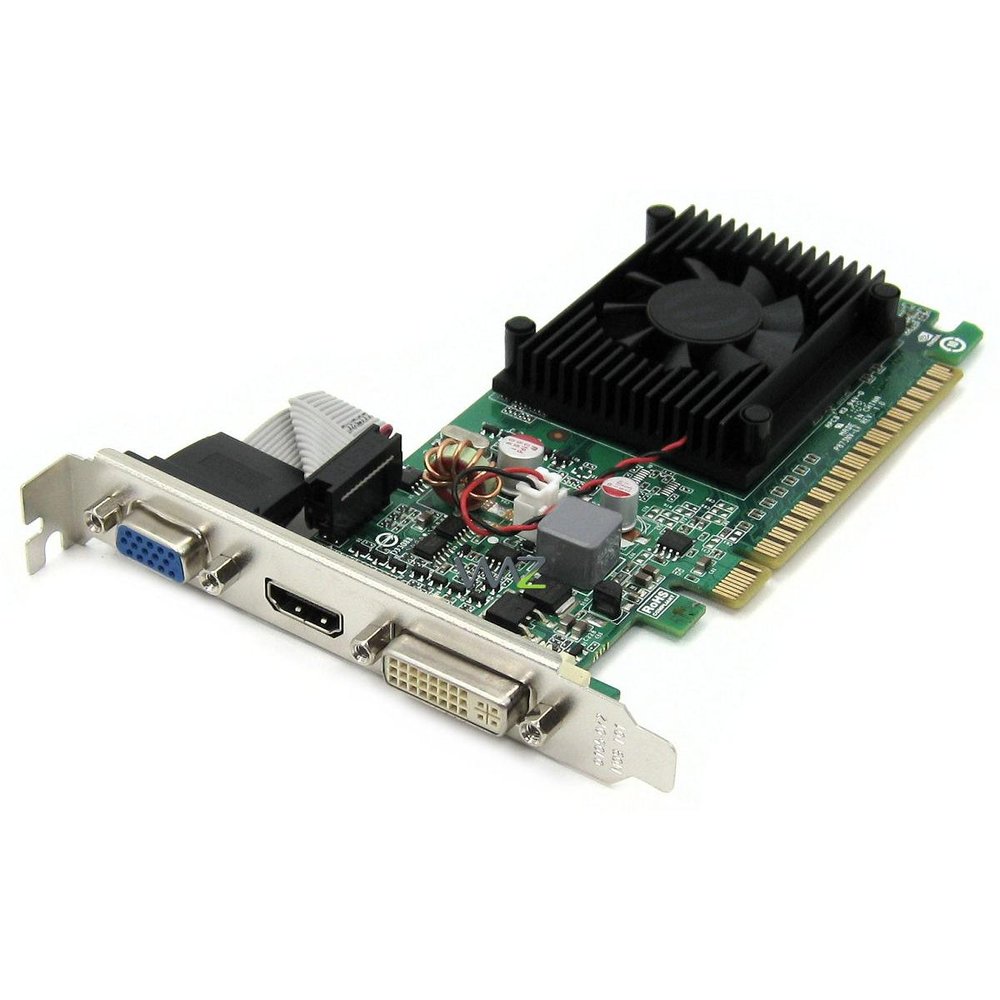
It was awesome to see this development and the application of these platforms to Deep Learning. During that process, I read a bit about GPUs, CUDA and cuDNN.
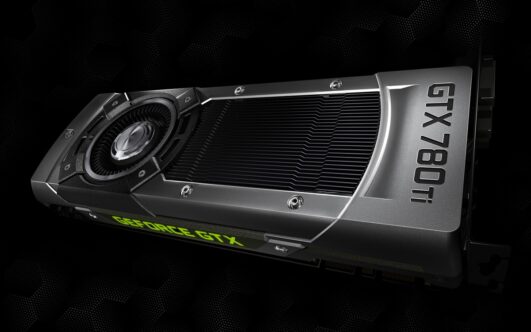
The only problem I encounter was to update the NVIDIA driver, and it was done easy. It was not a painful experience(as I was expecting) to use this hardware because Udacity provided an AIM with the necessary software already installed, and I didn’t need to install anything else. As part of the Udacity’s Self-Driving Car Nanodegree, I had the opportunity to try a GPU-powered server for Traffic Sign Classifier and the Behavioral Cloning projects in Term 1.
